Sensory Systems/Visual Color Perception
Color Perception
[edit | edit source]Introduction
[edit | edit source]Humans (together with primates like monkeys and gorillas) have the best color perception among mammals [1] . Hence, it is not a coincidence that color plays an important role in a wide variety of aspects. For example, color is useful for discriminating and differentiating objects, surfaces, natural scenery, and even faces [2],[3]. Color is also an important tool for nonverbal communication, including that of emotion [4].
For many decades, it has been a challenge to find the links between the physical properties of color and its perceptual qualities. Usually, these are studied under two different approaches: the behavioral response caused by color (also called psychophysics) and the actual physiological response caused by it [5].
Here we will only focus on the latter. The study of the physiological basis of color vision, about which practically nothing was known before the second half of the twentieth century, has advanced slowly and steadily since 1950. Important progress has been made in many areas, especially at the receptor level. Thanks to molecular biology methods, it has been possible to reveal previously unknown details concerning the genetic basis for the cone pigments. Furthermore, more and more cortical regions have been shown to be influenced by visual stimuli, although the correlation of color perception with wavelength-dependent physiology activity beyond the receptors is not so easy to discern [6].
In this chapter, we aim to explain the basics of the different processes of color perception along the visual path, from the retina in the eye to the visual cortex in the brain. For anatomical details, please refer to Sec. "Anatomy of the Visual System" of this Wikibook.
Color Perception at the Retina
[edit | edit source]All colors that can be discriminated by humans can be produced by the mixture of just three primary (basic) colors. Inspired by this idea of color mixing, it has been proposed that color is subserved by three classes of sensors, each having a maximal sensitivity to a different part of the visible spectrum [1]. It was first explicitly proposed in 1853 that there are three degrees of freedom in normal color matching [7]. This was later confirmed in 1886 [8] (with remarkably close results to recent studies [9], [10]).
These proposed color sensors are actually the so called cones (Note: In this chapter, we will only deal with cones. Rods contribute to vision only at low light levels. Although they are known to have an effect on color perception, their influence is very small and can be ignored here.) [11]. Cones are of the two types of photoreceptor cells found in the retina, with a significant concentration of them in the fovea. The Table below lists the three types of cone cells. These are distinguished by different types of rhodopsin pigment. Their corresponding absorption curves are shown in the Figure below.
Name | Higher sensitivity to color | Absorption curve peak [nm] |
---|---|---|
S, SWS, B | Blue | 420 |
M, MWS, G | Green | 530 |
L, LWS, R | Red | 560 |
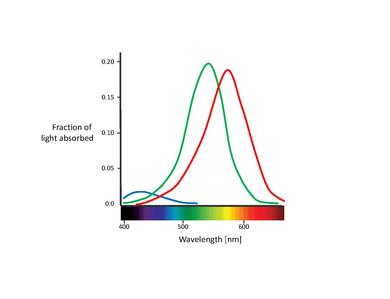
Although no consensus has been reached for naming the different cone types, the most widely utilized designations refer either to their action spectra peak or to the color to which they are sensitive themselves (red, green, blue)[6]. In this text, we will use the S-M-L designation (for short, medium, and long wavelength), since these names are more appropriately descriptive. The blue-green-red nomenclature is somewhat misleading, since all types of cones are sensitive to a large range of wavelengths.
An important feature about the three cone types is their relative distribution in the retina. It turns out that the S-cones present a relatively low concentration through the retina, being completely absent in the most central area of the fovea. Actually, they are too widely spaced to play an important role in spatial vision, although they are capable of mediating weak border perception [12]. The fovea is dominated by L- and M-cones. The proportion of the two latter is usually measured as a ratio. Different values have been reported for the L/M ratio, ranging from 0.67 [13] up to 2 [14], the latter being the most accepted. Why L-cones almost always outnumber the M-cones remains unclear. Surprisingly, the relative cone ratio has almost no significant impact on color vision. This clearly shows that the brain is plastic, capable of making sense out of whatever cone signals it receives [15], [16].
It is also important to note the overlapping of the L- and M-cone absorption spectra. While the S-cone absorption spectrum is clearly separated, the L- and M-cone peaks are only about 30 nm apart, their spectral curves significantly overlapping as well. This results in a high correlation in the photon catches of these two cone classes. This is explained by the fact that in order to achieve the highest possible acuity at the center of the fovea, the visual system treats L- and M-cones equally, not taking into account their absorption spectra. Therefore, any kind of difference leads to a deterioration of the luminance signal [17]. In other words, the small separation between L- and M-cone spectra might be interpreted as a compromise between the needs for high-contrast color vision and high acuity luminance vision. This is congruent with the lack of S-cones in the central part of the fovea, where visual acuity is highest. Furthermore, the close spacing of L- and M-cone absorption spectra might also be explained by their genetic origin. Both cone types are assumed to have evolved "recently" (about 35 million years ago) from a common ancestor, while the S-cones presumably split off from the ancestral receptor much earlier[11].
The spectral absorption functions of the three different types of cone cells are the hallmark of human color vision. This theory solved a long-known problem: although we can see millions of different colors (humans can distinguish between 7 and 10 million different colors[5], our retinas simply do not have enough space to accommodate an individual detector for every color at every retinal location.
From the Retina to the Brain
[edit | edit source]The signals that are transmitted from the retina to higher levels are not simple point-wise representations of the receptor signals, but rather consist of sophisticated combinations of the receptor signals. The objective of this section is to provide a brief of the paths that some of this information takes.
Once the optical image on the retina is transduced into chemical and electrical signals in the photoreceptors, the amplitude-modulated signals are converted into frequency-modulated representations at the ganglion-cell and higher levels. In these neural cells, the magnitude of the signal is represented in terms of the number of spikes of voltage per second fired by the cell rather than by the voltage difference across the cell membrane. In order to explain and represent the physiological properties of these cells, we will find the concept of receptive fields very useful.
A receptive field is a graphical representation of the area in the visual field to which a given cell responds. Additionally, the nature of the response is typically indicated for various regions in the receptive field. For example, we can consider the receptive field of a photoreceptor as a small circular area representing the size and location of that particular receptor's sensitivity in the visual field. The Figure below shows exemplary receptive fields for ganglion cells, typically in a center-surround antagonism. The left receptive field in the figure illustrates a positive central response (know as on-center). This kind of response is usually generated by a positive input from a single cone surrounded by a negative response generated from several neighboring cones. Therefore, the response of this ganglion cell would be made up of inputs from various cones with both positive and negative signs. In this way, the cell not only responds to points of light, but serves as an edge (or more correctly, a spot) detector. In analogy to the computer vision terminology, we can think of the ganglion cell responses as the output of a convolution with an edge-detector kernel. The right receptive field of in the figure illustrates a negative central response (know as off-center), which is equally likely. Usually, on-center and off-center cells will occur at the same spatial location, fed by the same photoreceptors, resulting in an enhanced dynamic range.
The lower Figure shows that in addition to spatial antagonism, ganglion cells can also have spectral opponency. For instance, the left part of the lower figure illustrates a red-green opponent response with the center fed by positive input from an L-cone and the surrounding fed by a negative input from M-cones. On the other hand, the right part of the lower figure illustrates the off-center version of this cell. Hence, before the visual information has even left the retina, processing has already occurred, with a profound effect on color appearance. There are other types and varieties of ganglion cell responses, but they all share these basic concepts.
On their way to the primary visual cortex, ganglion cell axons gather to form the optic nerve, which projects to the lateral geniculate nucleus (LGN) in the thalamus. Coding in the optic nerve is highly efficient, keeping the number of nerve fibers to a minimum (limited by the size of the optic nerve) and thereby also the size of the retinal blind spot as small as possible (approximately 5° wide by 7° high). Furthermore, the presented ganglion cells would have no response to uniform illumination, since the positive and negative areas are balanced. In other words, the transmitted signals are uncorrelated. For example, information from neighboring parts of natural scenes are highly correlated spatially and therefore highly predictable [18]. Lateral inhibition between neighboring retinal ganglion cells minimizes this spatial correlation, therefore improving efficiency. We can see this as a process of image compression carried out in the retina.
Given the overlapping of the L- and M-cone absorption spectra, their signals are also highly correlated. In this case, coding efficiency is improved by combining the cone signals in order to minimize said correlation. We can understand this more easily using Principal Component Analysis (PCA). PCA is a statistical method used to reduce the dimensionality of a given set of variables by transforming the original variables, to a set of new variables, the principal components (PCs). The first PC accounts for a maximal amount of total variance in the original variables, the second PC accounts for a maximal amount of variance that was not accounted for by the first component, and so on. In addition, PCs are linearly-independent and orthogonal to each other in the parameter space. PCA's main advantage is that only a few of the strongest PCs are enough to cover the vast majority of system variability [19]. This scheme has been used with the cone absorption functions [20] and even with the naturally occurring spectra[21],[22]. The PCs that were found in the space of cone excitations produced by natural objects are 1) a luminance axis where the L- and M-cone signals are added (L+M), 2) the difference of the L- and M-cone signals (L-M), and 3) a color axis where the S-cone signal is differenced with the sum of the L- and M-cone signals (S-(L+M)). These channels, derived from a mathematical/computational approach, coincide with the three retino-geniculate channels discovered in electrophysiological experiments [23],[24]. Using these mechanisms, visual redundant information is eliminated in the retina.
There are three channels of information that actually communicate this information from the retina through the ganglion cells to the LGN. They are different not only on their chromatic properties, but also in their anatomical substrate. These channels pose important limitations for basic color tasks, such as detection and discrimination.
In the first channel, the output of L- and M-cones is transmitted synergistically to diffuse bipolar cells and then to cells in the magnocellular layers (M-) of the LGN (not to be confused with the M-cones of the retina)[24]. The receptive fields of the M-cells are composed of a center and a surround, which are spatially antagonist. M-cells have high-contrast sensitivity for luminance stimuli, but they show no response at some combination of L-M opponent inputs[25]. However, because the null points of different M-cells vary slightly, the population response is never really zero. This property is actually passed on to cortical areas with predominant M-cell inputs[26].
The parvocellular pathway (P-) originates with the individual outputs from L- or M-cone to midget bipolar cells. These provide input to retinal P-cells[11]. In the fovea, the receptive field centers of P-cells are formed by single L- or M-cones. The structure of the P-cell receptive field surround is still debated. However, the most accepted theory states that the surround consists of a specific cone type, resulting in a spatially opponent receptive field for luminance stimuli[27]. Parvocellular layers contribute with about 80 % of the total projections from the retina to the LGN[28].
Finally, the recently discovered koniocellular pathway (K-) carries mostly signals from S-cones[29]. Groups of this type of cones project to special bipolar cells, which in turn provide input to specific small ganglion cells. These are usually not spatially opponent. The axons of the small ganglion cells project to thin layers of the LGN (adjacent to parvocellular layers)[30].
While the ganglion cells do terminate at the LGN (making synapses with LGN cells), there appears to be a one-to-one correspondence between ganglion cells and LGN cells. The LGN appears to act as a relay station for the signals. However, it probably serves some visual function, since there are neural projections from the cortex back to the LGN that could serve as some type of switching or adaptation feedback mechanism. The axons of LGN cells project to visual area one (V1) in the visual cortex in the occipital lobe.
Color Perception at the Brain
[edit | edit source]In the cortex, the projections from the magno-, parvo-, and koniocellular pathways end in different layers of the primary visual cortex. The magnocellular fibers innervate principally layer 4Cα and layer 6. Parvocellular neurons project mostly to 4Cβ, and layers 4A and 6. Koniocellular neurons terminate in the cytochrome oxidase (CO-) rich blobs in layers 1, 2, and 3[31].
Once in the visual cortex, the encoding of visual information becomes significantly more complex. In the same way the outputs of various photoreceptors are combined and compared to produce ganglion cell responses, the outputs of various LGN cells are compared and combined to produce cortical responses. As the signals advance further up in the cortical processing chain, this process repeats itself with a rapidly increasing level of complexity to the point that receptive fields begin to lose meaning. However, some functions and processes have been identified and studied in specific regions of the visual cortex.
In the V1 region (striate cortex), double opponent neurons - neurons that have their receptive fields both chromatically and spatially opposite with respect to the on/off regions of a single receptive field - compare color signals across the visual space [32]. They constitute between 5 to 10% of the cells in V1. Their coarse size and small percentage matches the poor spatial resolution of color vision [1]. Furthermore, they are not sensitive to the direction of moving stimuli (unlike some other V1 neurons) and, hence, unlikely to contribute to motion perception[33]. However, given their specialized receptive field structure, these kind of cells are the neural basis for color contrast effects, as well as an efficient mean to encode color itself[34],[35]. Other V1 cells respond to other types of stimuli, such as oriented edges, various spatial and temporal frequencies, particular spatial locations, and combinations of these features, among others. Additionally, we can find cells that linearly combine inputs from LGN cells as well as cells that perform nonlinear combination. These responses are needed to support advanced visual capabilities, such as color itself.

There is substantially less information on the chromatic properties of single neurons in V2 as compared to V1. On a first glance, it seems that there are no major differences of color coding in V1 and V2[36]. One exception to this is the emergence of a new class of color-complex cell[37]. Therefore, it has been suggested that V2 region is involved in the elaboration of hue. However, this is still very controversial and has not been confirmed.
Following the modular concept developed after the discovery of functional ocular dominance in V1, and considering the anatomical segregation between the P-, M-, and K-pathways (described in Sec. 3), it was suggested that a specialized system within the visual cortex devoted to the analysis of color information should exist[38]. V4 is the region that has historically attracted the most attention as the possible "color area" of the brain. This is because of an influential study that claimed that V4 contained 100 % of hue-selective cells[39]. However, this claim has been disputed by a number of subsequent studies, some even reporting that only 16 % of V4 neurons show hue tuning[40]. Currently, the most accepted concept is that V4 contributes not only to color, but to shape perception, visual attention, and stereopsis as well. Furthermore, recent studies have focused on other brain regions trying to find the "color area" of the brain, such as TEO[41] and PITd[42]. The relationship of these regions to each other is still debated. To reconcile the discussion, some use the term posterior inferior temporal (PIT) cortex to denote the region that includes V4, TEO, and PITd[1].
If the cortical response in V1, V2, and V4 cells is already a very complicated task, the level of complexity of complex visual responses in a network of approximately 30 visual zones is humongous. Figure 4 shows a small portion of the connectivity of the different cortical areas (not cells) that have been identified[43].
At this stage, it becomes exceedingly difficult to explain the function of singles cortical cells in simple terms. As a matter of fact, the function of a single cell might not have meaning since the representation of various perceptions must be distributed across collections of cells throughout the cortex.
Color Vision Adaptation Mechanisms
[edit | edit source]Although researchers have been trying to explain the processing of color signals in the human visual system, it is important to understand that color perception is not a fixed process. Actually, there are a variety of dynamic mechanisms that serve to optimize the visual response according to the viewing environment. Of particular relevance to color perception are the mechanisms of dark, light, and chromatic adaptation.
Dark Adaptation
[edit | edit source]Dark adaptation refers to the change in visual sensitivity that occurs when the level of illumination is decreased. The visual system response to reduced illumination is to become more sensitive, increasing its capacity to produce a meaningful visual response even when the light conditions are suboptimal[44].
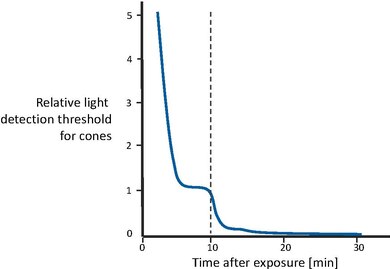
Figure 5 shows the recovery of visual sensitivity after transition from an extremely high illumination level to complete darkness[43]. First, the cones become gradually more sensitive, until the curve levels off after a couple of minutes. Then, after approximately 10 minutes have passed, visual sensitivity is roughly constant. At that point, the rod system, with a longer recovery time, has recovered enough sensitivity to outperform the cones and therefore recover control the overall sensitivity. Rod sensitivity gradually improves as well, until it becomes asymptotic after about 30 minutes. In other words, cones are responsible for the sensitivity recovery for the first 10 minutes. Afterwards, rods outperform the cones and gain full sensitivity after approximately 30 minutes.
This is only one of several neural mechanisms produced in order to adapt to the dark lightning conditions as good as possible. Some other neural mechanisms include the well-known pupil reflex, depletion and regeneration of photopigment, gain control in retinal cells and other higher-level mechanisms, and cognitive interpretation, among others.
Light Adaptation
[edit | edit source]Light adaptation is essentially the inverse process of dark adaptation. As a matter of fact, the underlying physiological mechanisms are the same for both processes. However, it is important to consider it separately since its visual properties differ.

Light adaptation occurs when the level of illumination is increased. Therefore, the visual system must become less sensitive in order to produce useful perceptions, given the fact that there is significantly more visible light available. The visual system has a limited output dynamic range available for the signals that produce our perceptions. However, the real world has illumination levels covering at least 10 orders of magnitude more. Fortunately, we rarely need to view the entire range of illumination levels at the same time.
At high light levels, adaptation is achieved by photopigment bleaching. This scales photon capture in the receptors and protects the cone response from saturating at bright backgrounds. The mechanisms of light adaptation occur primarily within the retina[45]. As a matter of fact, gain changes are largely cone-specific and adaptation pools signals over areas no larger than the diameter of individual cones[46],[47]. This points to a localization of light adaptation that may be as early as the receptors. However, there appears to be more than one site of sensitivity scaling. Some of the gain changes are extremely rapid, while others take seconds or even minutes to stabilize[48]. Usually, light adaptation takes around 5 minutes (six times faster than dark adaptation). This might point to the influence of post-receptive sites.
Figure 6 shows examples of light adaptation [43]. If we would use a single response function to map the large range of intensities into the visual system's output, then we would only have a very small range at our disposal for a given scene. It is clear that with such a response function, the perceived contrast of any given scene would be limited and visual sensitivity to changes would be severely degraded due to signal-to-noise issues. This case is shown by the dashed line. On the other hand, solid lines represent families of visual responses. These curves map the useful illumination range in any given scene into the full dynamic range of the visual output, thus resulting in the best possible visual perception for each situation. Light adaptation can be thought of as the process of sliding the visual response curve along the illumination level axis until the optimum level for the given viewing conditions is reached.
Chromatic Adaptation
[edit | edit source]The general concept of chromatic adaptation consists in the variation of the height of the three cone spectral responsivity curves. This adjustment arises because light adaptation occurs independently within each class of cone. A specific formulation of this hypothesis is known as the von Kries adaptation. This hypothesis states that the adaptation response takes place in each of the three cone types separately and is equivalent to multiplying their fixed spectral sensitivities by a scaling constant[49]. If the scaling weights (also known as von Kries coefficients) are inversely proportional to the absorption of light by each cone type (i.e. a lower absorption will require a larger coefficient), then von Kries scaling maintains a constant mean response within each cone class. This provides a simple yet powerful mechanism for maintaining the perceived color of objects despite changes in illumination. Under a number of different conditions, von Kries scaling provides a good account of the effects of light adaptation on color sensitivity and appearance[50],[51].
The easiest way to picture chromatic adaptation is by examining a white object under different types of illumination. For example, let's consider examining a piece of paper under daylight, fluorescent, and incandescent illumination. Daylight contains relatively far more short-wavelength energy than fluorescent light, and incandescent illumination contains relatively far more long-wavelength energy than fluorescent light. However, in spite of the different illumination conditions, the paper approximately retains its white appearance under all three light sources. This is because the S-cone system becomes relatively less sensitive under daylight (in order to compensate for the additional short-wavelength energy) and the L-cone system becomes relatively less sensitive under incandescent illumination (in order to compensate for the additional long-wavelength energy)[43].
References
[edit | edit source]- ↑ a b c d Conway, Bevil R (2009). "Color vision, cones, and color-coding in the cortex". The neuroscientist. 15: 274–290.
- ↑ Russell, Richard and Sinha, Pawan} (2007). "Real-world face recognition: The importance of surface reflectance properties". Perception. 36 (9).
{{cite journal}}
: CS1 maint: multiple names: authors list (link) - ↑ Gegenfurtner, Karl R and Rieger, Jochem (2000). "Sensory and cognitive contributions of color to the recognition of natural scenes". Current Biology. 10 (13): 805–808.
{{cite journal}}
: CS1 maint: multiple names: authors list (link) - ↑ Changizi, Mark A and Zhang, Qiong and Shimojo, Shinsuke (2006). "Bare skin, blood and the evolution of primate colour vision". Biology letters. 2 (2): 217–221.
{{cite journal}}
: CS1 maint: multiple names: authors list (link) - ↑ a b Beretta, Giordano (2000). Understanding Color. Hewlett-Packard.
- ↑ a b Boynton, Robert M (1988). "Color vision". Annual review of psychology. 39 (1): 69–100.
- ↑ Grassmann, Hermann (1853). "Zur theorie der farbenmischung". Annalen der Physik. 165 (5): 69–84.
- ↑ Konig, Arthur and Dieterici, Conrad (1886). "Die Grundempfindungen und ihre intensitats-Vertheilung im Spectrum". Koniglich Preussischen Akademie der Wissenschaften.
{{cite journal}}
: CS1 maint: multiple names: authors list (link) - ↑ Smith, Vivianne C and Pokorny, Joel (1975). "Spectral sensitivity of the foveal cone photopigments between 400 and 500 nm". Vision research. 15 (2): 161–171.
{{cite journal}}
: CS1 maint: multiple names: authors list (link) - ↑ Vos, JJ and Walraven, PL (1971). "On the derivation of the foveal receptor primaries". Vision Research. 11 (8): 799–818.
{{cite journal}}
: CS1 maint: multiple names: authors list (link) - ↑ a b c Gegenfurtner, Karl R and Kiper, Daniel C (2003). "Color vision". Neuroscience. 26 (1): 181.
{{cite journal}}
: CS1 maint: multiple names: authors list (link) - ↑ Kaiser, Peter K and Boynton, Robert M (1985). "Role of the blue mechanism in wavelength discrimination". Vision research. 125 (4): 523–529.
{{cite journal}}
: CS1 maint: multiple names: authors list (link) - ↑ Paulus, Walter and Kroger-Paulus, Angelika (1983). "A new concept of retinal colour coding". Vision research. 23 (5): 529–540.
{{cite journal}}
: CS1 maint: multiple names: authors list (link) - ↑ Nerger, Janice L and Cicerone, Carol M (1992). "The ratio of L cones to M cones in the human parafoveal retina". Vision research. 32 (5): 879–888.
{{cite journal}}
: CS1 maint: multiple names: authors list (link) - ↑ Neitz, Jay and Carroll, Joseph and Yamauchi, Yasuki and Neitz, Maureen and Williams, David R (2002). "Color perception is mediated by a plastic neural mechanism that is adjustable in adults". Neuron. 35 (4): 783–792.
{{cite journal}}
: CS1 maint: multiple names: authors list (link) - ↑ Jacobs, Gerald H and Williams, Gary A and Cahill, Hugh and Nathans, Jeremy (2007). "Emergence of novel color vision in mice engineered to express a human cone photopigment". Science. 315 (5819): 1723–1725.
{{cite journal}}
: CS1 maint: multiple names: authors list (link) - ↑ Osorio, D and Ruderman, DL and Cronin, TW (1998). "Estimation of errors in luminance signals encoded by primate retina resulting from sampling of natural images with red and green cones". JOSA A. 15 (1): 16–22.
{{cite journal}}
: CS1 maint: multiple names: authors list (link) - ↑ Kersten, Daniel (1987). "Predictability and redundancy of natural images". JOSA A. 4 (112): 2395–2400.
- ↑ Jolliffe, I. T. (2002). Principal Component Analysis. Springer.
- ↑ Buchsbaum, Gershon and Gottschalk, A (1983). "Trichromacy, opponent colours coding and optimum colour information transmission in the retina". Proceedings of the Royal society of London. Series B. Biological sciences. 220 (1218): 89–113.
{{cite journal}}
: CS1 maint: multiple names: authors list (link) - ↑ Zaidi, Qasim (1997). "Decorrelation of L-and M-cone signals". JOSA A. 14 (12): 3430–3431.
- ↑ Ruderman, Daniel L and Cronin, Thomas W and Chiao, Chuan-Chin (1998). "Statistics of cone responses to natural images: Implications for visual coding". JOSA A. 15 (8): 2036–2045.
{{cite journal}}
: CS1 maint: multiple names: authors list (link) - ↑ Lee, BB and Martin, PR and Valberg, A (1998). "The physiological basis of heterochromatic flicker photometry demonstrated in the ganglion cells of the macaque retina". The Journal of Physiology. 404 (1): 323–347.
{{cite journal}}
: CS1 maint: multiple names: authors list (link) - ↑ a b Derrington, Andrew M and Krauskopf, John and Lennie, Peter (1984). "Chromatic mechanisms in lateral geniculate nucleus of macaque". The Journal of Physiology. 357 (1): 241–265.
{{cite journal}}
: CS1 maint: multiple names: authors list (link) - ↑ Shapley, Robert (1990). "Visual sensitivity and parallel retinocortical channels". Annual review of psychology. 41 (1): 635--658.
- ↑ Dobkins, Karen R and Thiele, Alex and Albright, Thomas D (2000). "Comparison of red--green equiluminance points in humans and macaques: evidence for different L: M cone ratios between species". JOSA A. 17 (3): 545–556.
{{cite journal}}
: CS1 maint: multiple names: authors list (link) - ↑ Martin, Paul R and Lee, Barry B and White, Andrew JR and Solomon, Samuel G and Ruttiger, Lukas (2001). "Chromatic sensitivity of ganglion cells in the peripheral primate retina". Nature. 410 (6831): 933–936.
{{cite journal}}
: CS1 maint: multiple names: authors list (link) - ↑ Perry, VH and Oehler, R and Cowey, A (1984). "Retinal ganglion cells that project to the dorsal lateral geniculate nucleus in the macaque monkey". Neuroscience. 12 (4): 1101--1123.
{{cite journal}}
: Cite has empty unknown parameter:|month=
(help)CS1 maint: multiple names: authors list (link) - ↑ Casagrande, VA (1994). "A third parallel visual pathway to primate area V1". Trends in neurosciences. 17 (7): 305–310.
- ↑ Hendry, Stewart HC and Reid, R Clay (2000). "The koniocellular pathway in primate vision". Annual review of neuroscience. 23 (1): 127–153.
{{cite journal}}
: CS1 maint: multiple names: authors list (link) - ↑ Callaway, Edward M (1998). "Local circuits in primary visual cortex of the macaque monkey". Annual review of neuroscience. 21 (1): 47–74.
- ↑ Conway, Bevil R (2001). "Spatial structure of cone inputs to color cells in alert macaque primary visual cortex (V-1)". The Journal of Neuroscience. 21 (8): 2768–2783.
{{cite journal}}
: Cite has empty unknown parameter:|month=
(help) - ↑ Horwitz, Gregory D and Albright, Thomas D (2005). "Paucity of chromatic linear motion detectors in macaque V1". Journal of Vision. 5 (6).
{{cite journal}}
: CS1 maint: multiple names: authors list (link) - ↑ Danilova, Marina V and Mollon, JD (2006). "The comparison of spatially separated colours". Vision research. 46 (6): 823–836.
{{cite journal}}
: CS1 maint: multiple names: authors list (link) - ↑ Wachtler, Thomas and Sejnowski, Terrence J and Albright, Thomas D (2003). "Representation of color stimuli in awake macaque primary visual cortex". Neuron. 37 (4): 681–691.
{{cite journal}}
: CS1 maint: multiple names: authors list (link) - ↑ Solomon, Samuel G and Lennie, Peter (2005). "Chromatic gain controls in visual cortical neurons". The Journal of neuroscience. 25 (19): 4779–4792.
{{cite journal}}
: CS1 maint: multiple names: authors list (link) - ↑ Hubel, David H (1995). Eye, brain, and vision. Scientific American Library/Scientific American Books.
- ↑ Livingstone, Margaret S and Hubel, David H (1987). "Psychophysical evidence for separate channels for the perception of form, color, movement, and depth". The Journal of Neuroscience. 7 (11): 3416–3468.
{{cite journal}}
: CS1 maint: multiple names: authors list (link) - ↑ Zeki, Semir M (1973). "Colour coding in rhesus monkey prestriate cortex". Brain research. 53 (2): 422–427.
- ↑ Conway, Bevil R and Tsao, Doris Y (2006). "Color architecture in alert macaque cortex revealed by fMRI". Cerebral Cortex. 16 (11): 1604–1613.
{{cite journal}}
: CS1 maint: multiple names: authors list (link) - ↑ Tootell, Roger BH and Nelissen, Koen and Vanduffel, Wim and Orban, Guy A (2004). "Search for color 'center(s)'in macaque visual cortex". Cerebral Cortex. 14 (4): 353–363.
{{cite journal}}
: CS1 maint: multiple names: authors list (link) - ↑ Conway, Bevil R and Moeller, Sebastian and Tsao, Doris Y (2007). "Specialized color modules in macaque extrastriate cortex". 560--573. 56 (3): 560–573.
{{cite journal}}
: CS1 maint: multiple names: authors list (link) - ↑ a b c d Fairchild, Mark D (2013). Color appearance models. John Wiley & Sons.
- ↑ Webster, Michael A (1996). "Human colour perception and its adaptation". Network: Computation in Neural Systems. 7 (4): 587–634.
- ↑ Shapley, Robert and Enroth-Cugell, Christina (1984). "Visual adaptation and retinal gain controls". Progress in retinal research. 3: 263–346.
{{cite journal}}
: CS1 maint: multiple names: authors list (link) - ↑ Chaparro, A and Stromeyer III, CF and Chen, G and Kronauer, RE (1995). "Human cones appear to adapt at low light levels: Measurements on the red-green detection mechanism". Vision Research. 35 (22): 3103–3118.
{{cite journal}}
: CS1 maint: multiple names: authors list (link) - ↑ Macleod, Donald IA and Williams, David R and Makous, Walter (1992). "A visual nonlinearity fed by single cones". Vision research. 32 (2): 347–363.
{{cite journal}}
: CS1 maint: multiple names: authors list (link) - ↑ Hayhoe, Mary (1991). Adaptation mechanisms in color and brightness. Springer.
- ↑ MacAdam, DAvid L (1970). Sources of Color Science. MIT Press.
- ↑ Webster, Michael A and Mollon, JD (1995). "Colour constancy influenced by contrast adaptation". Nature. 373 (6516): 694–698.
{{cite journal}}
: CS1 maint: multiple names: authors list (link) - ↑ Brainard, David H and Wandell, Brian A (1992). "Asymmetric color matching: how color appearance depends on the illuminant". JOSA A. 9 (9): 1443–1448.
{{cite journal}}
: CS1 maint: multiple names: authors list (link)